Key Preview
Research Question
The main research question was to systematically examine the capabilities and limitations of large language models (LLMs), specifically GPT-3.5 and ChatGPT, in performing zero-shot medical evidence summarization across six clinical domains. This aimed to understand how well these models could handle the task of extracting and synthesizing key information from medical research studies and clinical trials.
Research Design and Strategy
The study designed two experimental setups. In one, the models were given the entire abstract (excluding the Author’s Conclusions) as input (ChatGPT-Abstract). In the other, they received both the Objectives and the Main Results sections of the abstract (ChatGPT-MainResult and GPT3.5-MainResult). The models were then prompted to summarize the review in four sentences, emphasizing the importance of referring to the Objectives section.
Method
The study utilized Cochrane Reviews from the Cochrane Library, focusing on six clinical domains. Two OpenAI models, GPT-3.5 and ChatGPT, were used. Automatic evaluation metrics such as ROUGE-L, METEOR, and BLEU were employed, along with a human evaluation that defined summary quality along four dimensions: coherence, factual consistency, comprehensiveness, and harmfulness.
Key Results
– Automatic Evaluation: All models had similar performance in automatic metrics. ROUGE score showed they could capture key information, but BLEU score indicated differences in writing style. LLMs were more extractive with lower n-gram novelty.
– Human Evaluation: ChatGPT-MainResult was more coherent and had fewer factual inconsistency errors. It also provided more comprehensive summaries. Harmfulness was lowest for ChatGPT-MainResult. In human preference, it was the most preferred as it included more salient information.
Significance of the Research
This research is crucial as it provides a comprehensive understanding of LLMs’ performance in medical evidence summarization. It highlights the need for better evaluation methods as automatic metrics may not be sufficient. The findings help in identifying areas where LLMs can be improved for reliable use in the medical field.
——————————————————————————————————————–
Introduction
Large language models have seen remarkable growth and have been applied in various text summarization tasks. Their ability to follow human instructions in a zero-shot setting has opened new possibilities. However, their application in medical evidence summarization is a novel area that requires careful evaluation. The complexity and importance of medical information demand accurate and reliable summarization. Challenges include ensuring factual consistency, comprehensiveness, and avoiding potential harm due to misinformation.
Research Objective
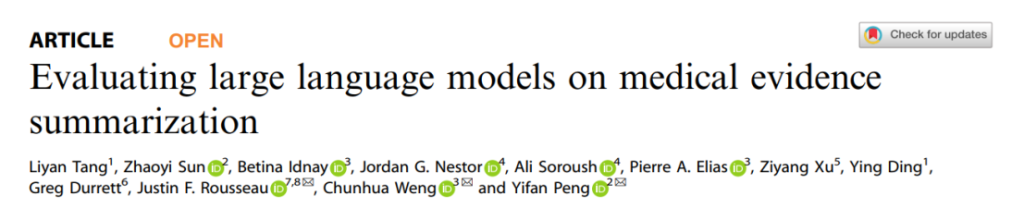
The research was conducted by Liyan Tang, Zhaoyi Sun, and others from institutions like The University of Texas at Austin and Columbia University. The paper “Evaluating large language models on medical evidence summarization” was published in npj Digital Medicine in 2023. The significance lies in understanding the potential and limitations of LLMs in a critical domain. The objective was to assess how well these models could summarize medical evidence to assist healthcare professionals in making informed decisions.
Experimental Process
Data Collection
Cochrane Reviews from the Cochrane Library were used, focusing on six clinical domains. Ten reviews (except for some domains with fewer) were collected for each domain. Domain experts verified the reviews.
Model Input Setups
– ChatGPT-Abstract: Models took the whole abstract except the Author’s Conclusions.
– ChatGPT-MainResult and GPT3.5-MainResult: Models received the Objectives and Main Results sections.
Prompting Method
The prompt was [input] + “Based on the Objectives, summarize the above systematic review in four sentences”.
Results and Significance
1. Automatic Evaluation Results: ROUGE-L values were relatively high, indicating key information capture. For example, ChatGPT-MainResult had a certain ROUGE-L score. BLEU scores were low, showing differences in writing. METEOR scores were consistent. Extractiveness metrics showed LLMs were more extractive.
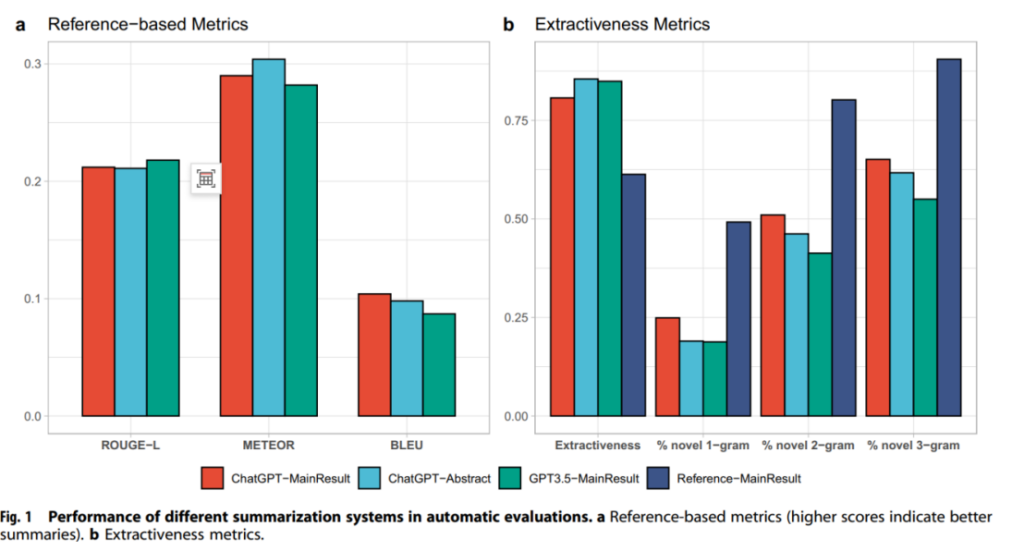
- Human Evaluation Results: In coherence, ChatGPT-MainResult had a higher percentage of strong agreement. For factual consistency, it had fewer errors. In comprehensiveness, it provided more comprehensive summaries. In harmfulness, it generated the fewest medically harmful summaries.
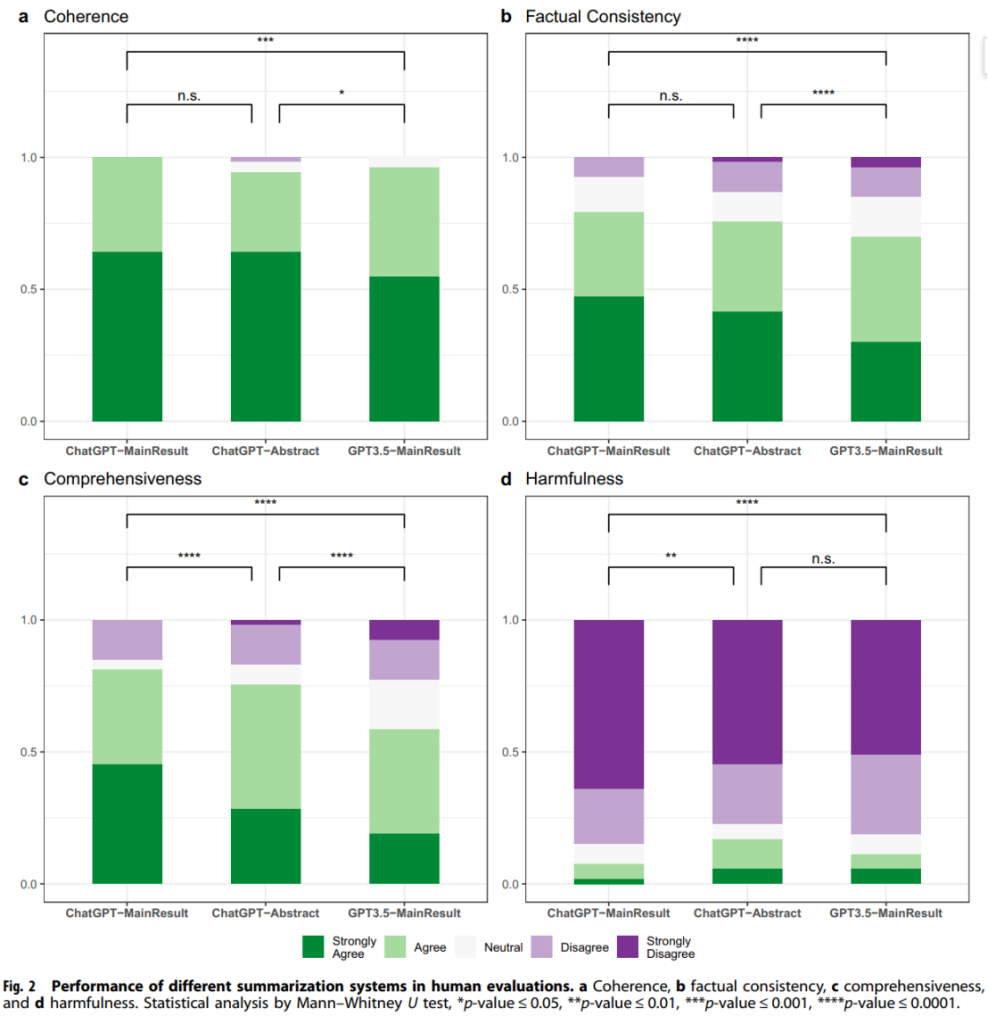
– Significance: These results show the varying capabilities of LLMs. The differences in performance highlight the need for better model training and input design. The new error type definitions in human evaluation provide a way to understand and address the issues in summarization.
Conclusion
Key Findings
LLMs like GPT-3.5 and ChatGPT showed mixed performance. They had some ability to capture key information but also had issues with factual consistency, making errors like misinterpretation (including contradiction and certainty illusion), fabricated errors (though rare for ChatGPT), and attribute errors. ChatGPT-MainResult generally performed better in human evaluations.
Implications
The findings suggest that while LLMs have potential, they need improvement before being reliably used for medical evidence summarization. The risk of misinformation and harm due to inaccurate summaries is a concern.
Limitations
The study used a semi-synthetic task and the prompt was adapted from previous work. The evaluation was limited by the use of only abstracts and a small number of annotators.
Future Research Suggestions
Future work could explore GPT-4 and develop more effective evaluation methods. It should also focus on improving the models’ ability to handle longer contexts and detect factual inconsistencies and medical harmfulness.
——————————————————————————————————————–
Reference: Tang, Liyan, et al. “Evaluating large language models on medical evidence summarization.” NPJ digital medicine 6.1 (2023): 158.