A recent study systematically examines the capabilities and limitations of large language models, specifically GPT-3.5 and ChatGPT, in performing zero-shot medical evidence summarization across six clinical domains, revealing potential risks of misinformation and the struggle to identify salient information.
Key Highlights
Research Question: The study aims to evaluate the zero-shot performance of large language models (LLMs), specifically GPT-3.5 and ChatGPT, in summarizing medical evidence across various clinical domains and to understand their potential limitations.
Research Difficulties: Challenges included the lack of sizable training datasets in medical domains, the high stakes of accurate information in clinical settings, and the need for models to follow human instructions without updating parameters.
Key Findings: LLMs are susceptible to generating factually inconsistent summaries and making overly convincing or uncertain statements, which could lead to potential harm due to misinformation. Models struggle to identify salient information and are more error-prone when summarizing over longer textual contexts.
Innovative Aspects: The study introduces a terminology of error types for medical evidence summarization and conducts both automatic and human evaluations to assess summary quality across several dimensions.
Importance of the Study: The findings are crucial for understanding the reliability and potential risks of using LLMs in high-stakes medical domains where misinformation can have serious consequences. ],
The Challenge of Medical Evidence Summarization
Medical evidence summarization is a critical process for extracting and synthesizing key information from a vast array of medical research studies and clinical trials into concise and comprehensive summaries. This process is essential for healthcare professionals to make informed decisions about treatment options. Current challenges include the lack of training data in medical domains and the need for summaries that are accurate, comprehensive, and coherent.
Decoding LLMs’ Performance in Medical Evidence Summarization
The study aimed to conduct a systematic evaluation of the potential and limitations of zero-shot prompt-based LLMs on medical evidence summarization using GPT-3.5 and ChatGPT models. The goal was to assess their impact on the summarization of medical evidence findings in the context of evidence synthesis and meta-analysis. The research objectives were to evaluate the models’ capabilities in extracting key information, maintaining factual consistency, and generating summaries that are coherent and comprehensive.
Evaluating LLMs’ Capabilities in Medical Evidence Summarization
The study utilized Cochrane Reviews from the Cochrane Library, focusing on six clinical domains: Alzheimer’s disease, Kidney disease, Esophageal cancer, Neurological conditions, Skin disorders, and Heart failure. The experimental process involved two distinct setups: one where models were given the entire abstract excluding the Author’s Conclusions (ChatGPT-Abstract), and another where models received both the Objectives and the Main Results sections from the abstract as input (ChatGPT-MainResult and GPT3.5-MainResult).
Key Experiment 1: Single-Document Summarization – The models were tasked with summarizing the abstracts of Cochrane Reviews, which are standalone documents containing key methods, results, and conclusions of the review. The prompt used was ‘[input] + “Based on the Objectives, summarize the above systematic review in four sentences”’. The average word count of the generated summaries was 107, 111, and 95 for ChatGPT-MainResult, ChatGPT-Abstract, and GPT3.5-MainResult, respectively.
Results: The automatic evaluation using metrics such as ROUGE-L, METEOR, and BLEU revealed similar performance across all models, with high ROUGE scores indicating effective key information capture but low BLEU scores. Human evaluations, however, showed that ChatGPT-MainResult generated the most preferred summaries, with fewer factual inconsistency errors and less potential for medical harmfulness.
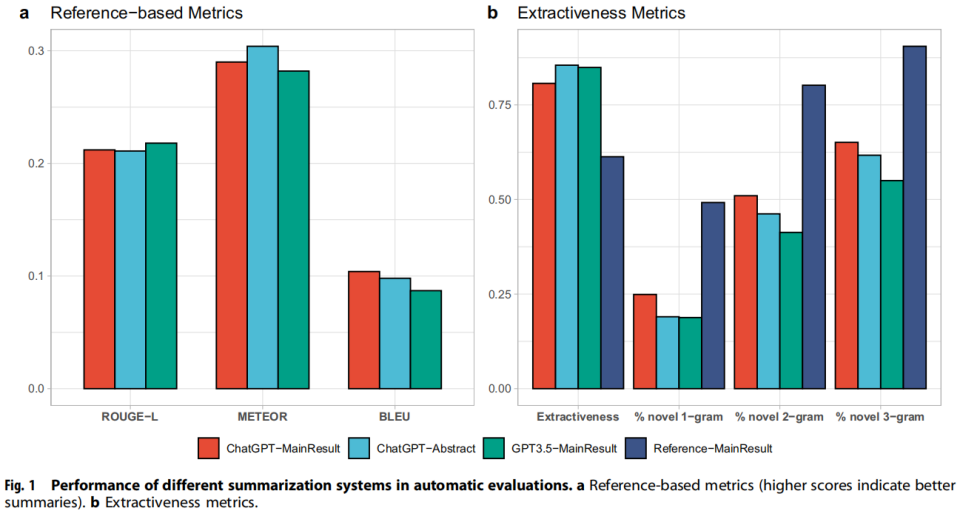
Key Experiment 2: Error Analysis and Human Evaluation – Human evaluators assessed the summaries along four dimensions: Coherence, Factual Consistency, Comprehensiveness, and Harmfulness. The evaluation revealed that LLMs struggle with identifying salient information and are prone to making factually inconsistent statements, which could lead to misinformation and potential harm.
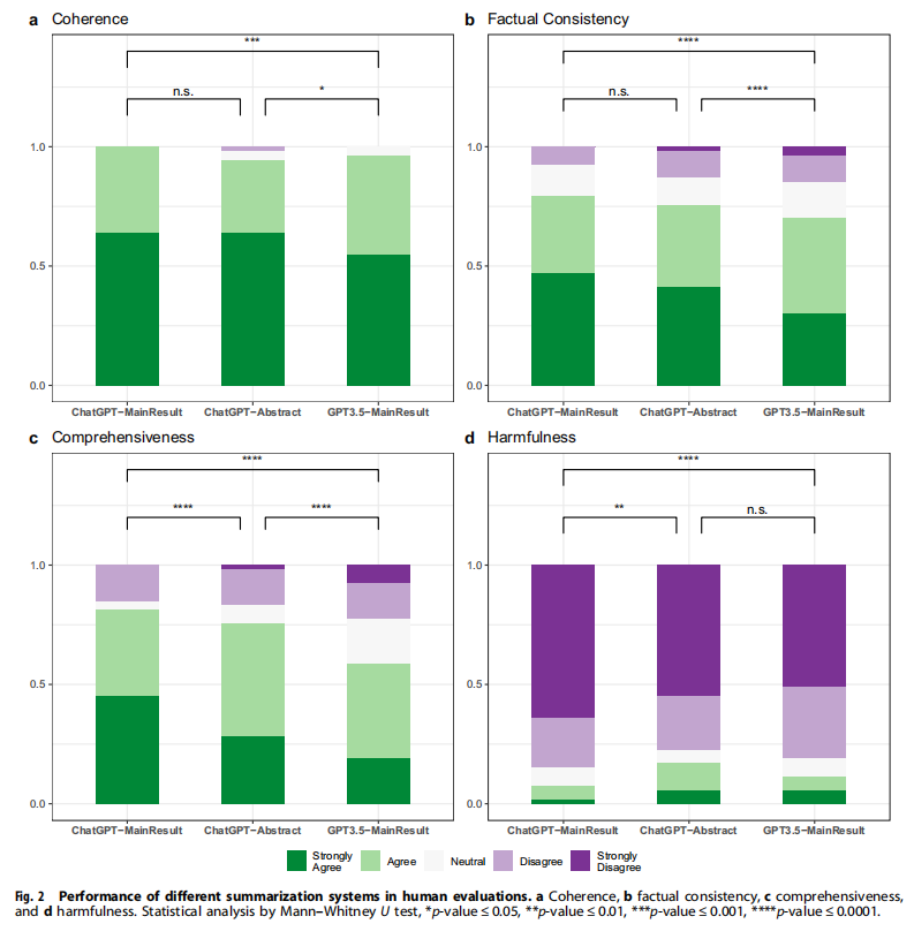
Significance: The findings suggest that while LLMs can generate summaries that capture key information, they often fail to maintain factual consistency and may generate summaries that are potentially harmful due to misinformation. This has significant implications for the use of LLMs in high-stakes medical domains.
Synthesizing the Findings and Their Implications
The study’s findings indicate that large language models, despite their ability to capture key information, often fail to maintain factual consistency and may generate summaries that are potentially harmful due to misinformation. The research introduces a terminology of error types for medical evidence summarization and demonstrates the need for both automatic and human evaluations to assess summary quality. The innovation of this study lies in its systematic evaluation of LLMs in a high-stakes domain and the development of a framework for assessing the quality and factuality of medical evidence summaries.
Reference:
Tang, Liyan, et al. “Evaluating large language models on medical evidence summarization.” NPJ digital medicine 6.1 (2023): 158.